MAPS
Machine learning and Algorithms for Practical Security
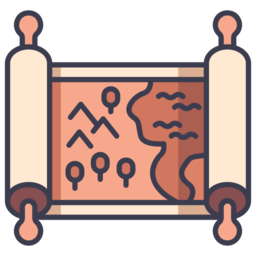
Machine learning tools have become widely accessible over the past decade, but their security remains an ongoing challenge. OWASP has summarized the ‘Top 10’ practical problems in machine learning (ML) security. However, research in each sub-problem is an ongoing race between attacks and defenses. Does this cat-and-mouse race have an end? Are there optimal defense strategies such that all attacks bounded by certain costs become impractical?
The MAPS project aims to answer these questions in a principled manner by identifying the inherent limitations of current schemes and drawing from cryptographically hard problems to establish robust security guarantees. Specifically, we study four main areas:
- the practical impact of data poisoning attacks in federated settings and the computational limitations of robust aggregation defenses against such attacks;
- watermarking schemes for AI-generated content that is provably secure against all possible attacks;
- we investigate verification of desired properties of ML systems and practical differential privacy in federated networks and GNNs.
MAPS is a project under the KISP NUS Lab.
-
Poisoning Attacks & Robust Aggregation
Data poisoning attack is when an attacker corrupts training data to cause undesirable behaviour in the trained model. This behaviour can be of various kinds, including but not limited to inserting backdoors, decreasing overall accuracy, misclassifying certain inputs, and so on. [Read More] -
Watermarking AI
Protection of intellectual property and verifying data authenticity is a long-existing problem. In the presence of multiple generative AI frameworks, a question naturally arises on how can we verify the source of a text/image/sound data sample? One solution is watermarking. However, watermarking should not affect downstream tasks. This calls for... [Read More] -
Others
We also present principled approaches to analyzing machine learning security in many other aspects, such as robust verification and privacy. For example, we applied quantitative verification to check fairness and adversarial robustness of neural networks, in a sound and scalable manner. We also analyzed the root causes of membership inference... [Read More]
Publications
Recent
Current members
We gratefully acknowledge the support of Crystal Center.